Waylay has been in business since 2014 and from meeting with equipment manufacturers across the world, we have identified 5 key learnings to put IIoT data to better operational use.
It is obvious today that IIoT facilitates the transition to optimized after-sales support and service based business models, but that will only work if done right and if the following five statements are taken into consideration.
- Move beyond dashboard monitoring
- Look beyond R&D for content creation
- Take a results oriented approach to analytics & AI
- Be aware of reality of scattered information
- Not all assets are connected from day one
The IIoT should help product companies to offer outcome-based service contracts and to move away from one-off sales and their break-&-fix approach. Equipment manufacturers will migrate to new business models that rely on access to digital twin data that continuously monitor the equipment and offer a service contract with a guaranteed service level and quality.
Waylay has been working with equipment manufacturers for years and we see that IIoT is the enabler to help these companies to optimize their after sales support and from this experience, Waylay has detected a number of pitfalls and dependencies to make this shift towards outcome-based service models successful.
1. Move beyond dashboard monitoring
IIoT data provide valuable insights but to get to its full exploit, equipment manufacturers need to move beyond dashboard monitoring. Data is a company’s crude oil, but needs refining to extract its true gold. The first step is collecting data, visualizing it, building dashboards and creating alarms in order to provide tech people with access and visibility on what is happening with the equipment. In a second step, companies will want to share these data with other stakeholders within the company, with external customers and with partners. Data needs to be enriched so that the right set of people get access to the relevant set of data and can be shared via integration with other systems. In the next step, equipment manufacturers will want to further optimize after-sales support through the use of IIoT data. This is the domain where analytics, prediction algorithm and anomaly detection play a key role. And finally companies will start using “as a Service” business models which are not only depending on technology, but also imply a complete overhaul of the go-to-market of equipment manufacturers and their customers. In short, dashboard monitoring is indeed the first step, but also only the first step to unfold the full potential of IIoT data.
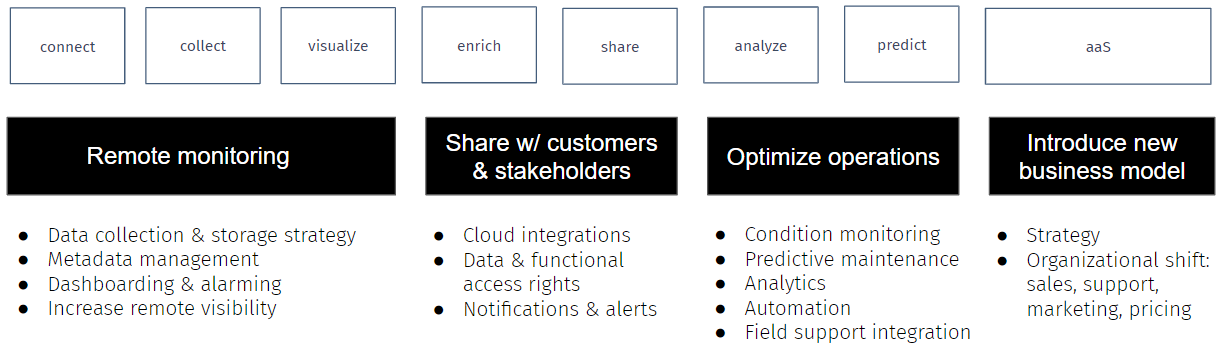
2. Look beyond software R&D for content creation
Data scientists and domain experts know a lot about their equipment, but cannot fully take advantage of their expertise as they have to funnel their ideas and proposals through their software R&D teams. In a standard R&D cycle, field technicians, domain experts, data scientists as well as the final customers have their own ideas on how the product could or should evolve. All the different requirements and expectations will be pushed into a classical software R&D funnel which is often driven and owned by internal IT partners and external IT parties. These requirements will go through an iterative development cycle that typically takes some 6 months before a new release or new product is ready.
Today Waylay offers a fast-track alternative with their low code approach which allows non-software developers to also create content: data scientists and domain experts can add their own algorithms and analytics without having to rely on a full R&D cycle, which leads to a much faster time to market and democratization of analytics capabilities within every organization.
3. Take a results oriented approach to analytics & AI
Companies wanting to exploit their data, should not only look at analytics and AI from a technology perspective, but instead start from the use case and use AI to get to the desired result. And this reversed concept holds two components that are not necessarily sequential steps, but often happen at the same time.
First, brilliant data scientists can develop analytics or machine learning algorithms based on their favorite toolstack. But next, the question arises on how to make the algorithms operational, how to test different hypotheses and how to apply the algorithms on large sets of data. This is where data scientists often get stuck, as they need a software development cycle and a lot of patience to experiment with their algorithms and fine-tune the business cases. With Waylay, data scientists can integrate their algorithms into the automation flows, no matter how the algorithm was developed.
In addition, to obtain the best results in analytics, companies need to combine domain expertise, their deep knowledge of equipment and physical processes with analytics algorithms. Not just one or the other, but a combination of both. Waylay helps to express these heuristics with a holistic approach and embeds the algorithm for best results in any specific use case.
4. Be aware of reality of scattered information
Often we think of IIoT as a greenfield situation, but in real life it is brownfield and businesses need to combine data from different sources to get to the right outcome.
Obviously, data sources are diverse and can originate from IIoT, OT and IT information.
Sensors or IIoT gateways provide large amounts of data that live in IIoT platforms, IT environments collect information from ERP systems and asset management systems, OT gathers data through SCADA systems and finally, the data influx from analytics and machine learning algorithms can complete the IIoT data abundance. Only if we can ingest, process and combine this plethora of data in a flexible and automated way, we can generate relevant and actionable results.
5. Not all assets are connected from day 1
In an ideal world, all assets would be connected, but in reality that is often not the case (yet) at the start of an IIoT trajectory. However, there is definitely real value, that can be extracted, for the assets not yet connected. For assets that are disconnected, log files can be uploaded periodically as they can be retrieved from the equipment and fed into the system for further processing. So next to streaming processing for connected equipment, there is also the need to work with batch processing data for non-connected equipment where the same business logic can be applied for both sets of data. From then, equipment manufacturers will have a value proposition for both connected as well as non-connected devices.
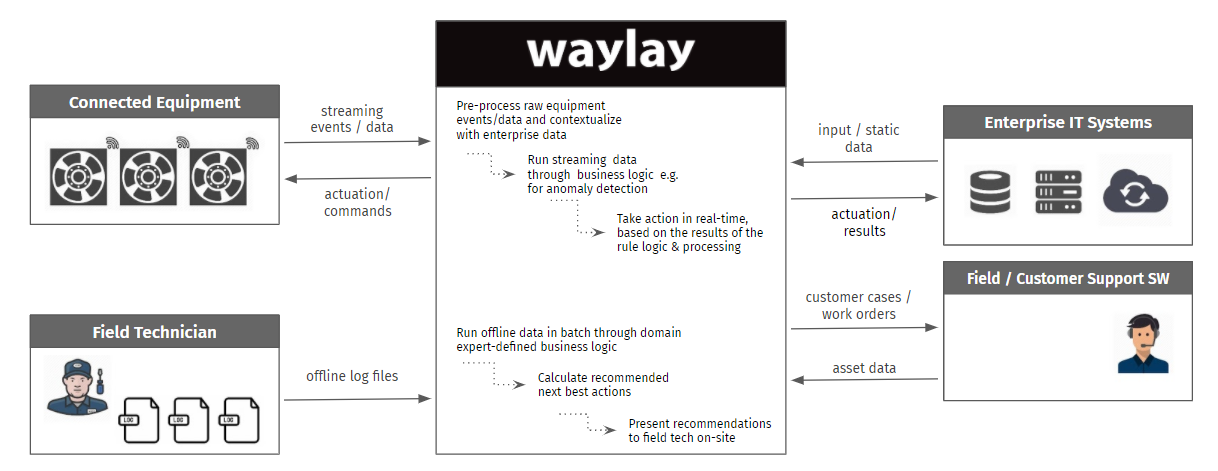
Conclusion
We will see the world evolving from one-off product sales to outcome-based business models in the next few years. IIoT is a great enabler for new business models, if done right and Waylay empowers organizations to focus on concrete business value and outcomes rather than low level IT details. It empowers field technicians, data scientists and domain experts to express their domain knowledge through novel analytics algorithms and allows them to experiment with and discover the real value of their data. Via integration of IIoT, OT and IT, Waylay enables optimized processes through integration with the equipment manufacturers’ specific line of business applications. Combining these five learnings empowers equipment manufacturers to get more out of the IIoT data they collect. Equipment manufacturers enable the domain experts to productize their domain knowledge in an efficient way and fast, in a matter of just weeks, rather than a development cycle of several months.
Waylay provides the data processing technology that allows companies to do more with the data they collect, through a low code development approach that brings new use cases to market quickly and at a competitive TCO.